Gary Frost put it nicely when he described self-reported energy assessments as the Achilles heel of nutrition science.
The difficulties with dietary assessments
During Gary Frost’s talk “Improving the accuracy of dietary intake assessment: From molecules to cameras” given at the Nordic Nutrition Conference 2020, he mentioned how many fantastic scientific breakthroughs we’ve had during the last 60-70 years, and yet, we have not figured out how to accurately assess what people eat in their day to day life.
Traditional dietary assessment methods including food questionnaire’s and dietary recalls rely on self-reported dietary data. During the 1980s, doubly-labelled water studies (an objective method determining a person’s energy expenditure) highlighted that under-reporting of energy intake was very common with self-reported diet assessment methods (1). Consequently, use of objective diet assessment methods is of interest. Using doubly-labelled water is however quite costly and may therefore not be suitable for population-wide use.
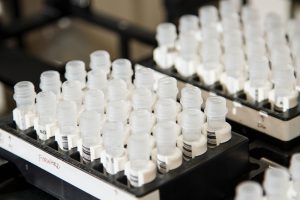
Metabolomics
As a promising method for tackling the inherent issues of self-reported diet assessment methods, Gary Frost brings up metabolomics. Specifically, analysing urine samples using Nuclear Magnetic Resonance (NMR) spectroscopy and based on the output identify a metabolic profile (with individual molecules being markers for specific foods). He presented results from a randomised controlled trial with 19 individuals, in which researchers were able to identify distinct dietary patterns based on the metabolic profiles (3). Interestingly, they were able to clearly separate individuals with ‘healthy’ and ‘unhealthy’ diets using this method. This metabolomic profiling system has also been used in free-living participants in cohorts, for example Intermap UK, where adherence to the DASH diet signified healthy eaters (3).
Further, Gary Frost presented results from a clinical trial showing how metabolomic phenotypes can be used to predict responses to dietary interventions. He highlighted two participants who responded in the same way to a diet with regard to glucose control, but that they got there using very different metabolic pathways (4). In the future, Gary Frost predicted that metabolomics will be utilised in clinical practice as well as epidemiological studies to identify metabolomic profiles and metabolic pathways as the method is relatively fast and inexpensive.
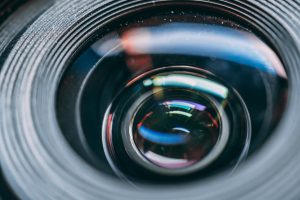
Camera technologies
Although metabolomics provide a lot of insight, it has its limitations. Certain components of the diet are currently ‘invisible’ for the metabolomic technologies, for example energy and carbohydrate intake. Along with developing metabolomics technologies, Gary Frost suggests combining it with other methods. One such method that his team is currently studying is passive dietary monitoring using imaging technologies.
The idea is to, using machine learning and artificial intelligence, identify eating episodes, food types, portion sizes, and nutritional information solely by passive (often wearable) camera monitoring. There are several ongoing studies testing these technologies in The UK, Ghana, and Kenya. So far, the results indicate that the technology provides a good estimation of weight of food when compared with direct weighing or estimations done by dieticians. However, there are many challenges, including difficulties estimating intake of shared foods and privacy concerns. There seems to be many hurdles to overcome before being able to use these passive camera technologies for diet assessment, but they could potentially evolve to become a tool to offer full nutritional insight!
References
1. Schoeller DA, Bandini LG, Dietz WH. Inaccuracies in self-reported intake identified by comparison with the doubly labelled water method. Can J Physiol Pharmacol. 1990;68(7):941-9.
2. Ramne S, Gray N, Hellstrand S, Brunkwall L, Enhorning S, Nilsson PM, et al. Comparing Self-Reported Sugar Intake With the Sucrose and Fructose Biomarker From Overnight Urine Samples in Relation to Cardiometabolic Risk Factors. Front Nutr. 2020;7:62.
3. Garcia-Perez I, Posma JM, Gibson R, Chambers ES, Hansen TH, Vestergaard H, et al. Objective assessment of dietary patterns by use of metabolic phenotyping: a randomised, controlled, crossover trial. The Lancet Diabetes & Endocrinology. 2017;5(3):184-95.
4. Garcia-Perez I, Posma JM, Chambers ES, Mathers JC, Draper J, Beckmann M, et al. Dietary metabotype modelling predicts individual responses to dietary interventions. Nature Food. 2020;1(6):355-64.